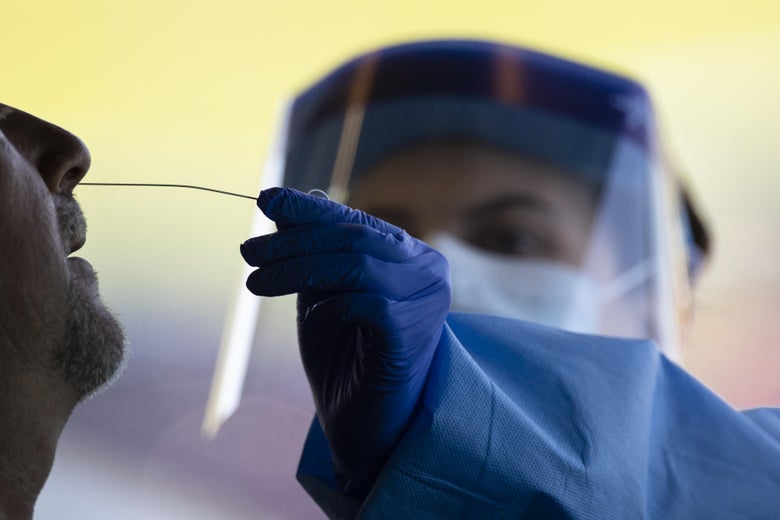
Testing sites like this one in Springfield, Tennessee, photographed April 18, can only catch so many cases.
Brett Carlsen/Getty Images
As of Monday afternoon, there have now been more than 3 million confirmed cases of COVID-19 and more than 200,000 recorded deaths worldwide, one-quarter of which have been in the United States.
But we don’t really know how many people have been infected or how fast the disease is spreading, because existing tests on selected groups of people don’t give a sense of the prevalence of the coronavirus in the general population. (Here’s a quick explainer on the need for global testing for the virus and its antibodies, and how these tests work.)
In addition to directly testing for the virus in representative populations, we can and should be watching for symptoms. Learning about the prevalence of symptoms is valuable for at least four reasons. First, this is a new disease, and we want to learn about its symptoms, both for diagnosis and for treatment. Second, symptoms give us an estimate of disease prevalence (after adjusting for unavoidable reporting errors). Third, projecting symptom rates should help national, state, and local authorities better manage resources such as hospital beds. Fourth, tracking changes in symptom rates over time in different places can help us learn about the spread of the epidemic and estimate the effects of various containment strategies.
Without better data, the much-discussed coronavirus models are all inherently speculative. But researchers are working to change that—including with a large new study of coronavirus symptoms being conducted by a team at Carnegie Mellon University, led by computer scientist Roni Rosenfeld and statistician Ryan Tibshirani. The group has been working for several years on influenza tracking and recently expanded to study the pandemic. The public-facing side of the project is COVIDcast, a set of daily-updated real-time updates at the county level. (More background here.)
The idea of this new study is to track symptoms, not infections or deaths, and to use this information to predict hospitalizations. These time trends and forecasts are relevant for informing state and local policies and recommendations that can change flexibly over time.
One distinguishing feature of the CMU study that it aggregates data from five sources. The first two are surveys of self-reported symptoms run daily through Google and Facebook, which have been receiving hundreds of thousands of responses. The surveys on the two platforms have different sampling models, which are somewhat complementary. Right now, about 0.9 percent of respondents to the Facebook survey are self-reporting symptoms, and the number stays at about this level after they make adjustments for nonresponse by state, age, and sex.
Next there’s a survey of outpatient visits that come from a national health system, and it’s based off of real-time access to insurance claim statistics that can be used to estimate the percentage of patient visits that COVID-related.
The fourth signal is based on search trends on Google. That surprised me. When Google trends for the flu first came out a few years ago, there was a lot of press, a lot of hype. And then I thought there was a point where people said it didn’t really work like it was advertised. (In 2015, Wired called Google Flu Trends an “epic failure.”) I asked Rosenfeld and Tibshirani about this and they said that, yes, the raw numbers from web searches can be misleading, but if you consider search trends as predictors in combination with other data, and you repeatedly calibrate with new data, the trends can be informative.
Their fifth signal is based off of flu tests—yes, flu, not coronavirus. They are working with a company called Quidel, which makes diagnostics and is giving real-time access to its data. Rosenfeld and Tibshirani have found that that the prevalence of flu testing is correlated with COVID activity. That’s because when people come in with COVID symptoms or otherwise suspect it, one of the standard things that are being done now is testing for flu, because the test is available and well understood, and we want to rule out flu. The symptoms are highly overlapping.
Those five signals cover a lot of ground. The big concern with all these sources of information, though, is that the path of the pandemic keeps changing, maybe behaving in a way that old predictions can become quickly irrelevant. But that’s true even with seasonal flu. Rosenfeld and Tibshirani found that if you use Google search trends in a way that properly kind of accounts for system changes over time (what statisticians call “nonstationarity”), then it still provides value when combined with many other sources.
Rosenfeld and Tibshirani say that their team can’t really make the raw data available because it’s confidential survey responses, insurance information, and so forth, but they plan to release aggregate estimates of symptom rates by county from each of the data sources, and to put these together to come up with predictions of future hospitalization rates at the level of local health care systems (regions that are typically between 10 and 15 counties each). The important thing about the hospitalization forecasts is that they can be checked each week as new data come in. The CMU team can use this to correct biases and train its prediction model going forward.
“We’ve done the best job we can do in the kind of time we had to make sense of these data sources. We’ve run analyses to internally to kind of check that they are not garbage, that they correlate with the things we expect them to correlate with,” Tibshirani says. “The path forward after this would be to make forecasts based on them and then probably at the same time to try to do ‘nowcasting’ in order to make some statement about this is what we think the current state is in the U.S.”
The most important use for this information may be to make fine-tuned decisions about loosening or tightening mitigation measures (like social distancing) in individual regions of the country. “You want to re-open the local economy as much as possible without exceeding the local acute care capacity (ICU beds, ventilators, trained personnel to operate them),” Rosenfeld writes. “Changes to mitigation measures, in either direction, affect demand on hospitals within 2-3 weeks, hence the forecasting timeframe we focus on, and our accommodation of different possible mitigation scenarios into our forecasts.”
The goals of this project are a bit different from some of the other forecasts we have been hearing about. Estimation of key epidemiological parameters is important in its own right, to understand the dynamic of the pandemic, learn from it, and devise long term-strategies. The goal of Rosenfeld and Tibshirani’s symptom and hospitalization monitoring program, on the other hand, is very tactical: They focus on short-term forecasting, for immediate decision making. They care less about the true value of these key quantities and more on what inputs and features are most predictive of the targets we care about. They focus on the technology of forecasting epidemics, much more so than on the science of epidemiology. There is, of course, great need for both.
I asked Rosenfeld what his recommendations are for policymakers based on what the CMU team’s work has found so far. “First, bring together the largest health care organizations in the country and urge them to share their anonymized data into a centralized repository. This is by far the most important type of data for both situational awareness and forecasting,” he said. “Second, don’t rely on any particular model, especially with regard to long term predictions. Only trust verifiable predictions with a track record of accuracy. Third, in the spirit of not relying on models: make short-term reversible decisions on gradual opening up of parts of the economy in parts of the country. Keep your eyes on the local pulse of the epidemic. As you move along, keep track of the accuracy of different short-term forecasts.”
These sorts of continuous data-collection, forecasting, and calibration projects will have value long after the pandemic has faded. As a society, we should be monitoring our public health with the same care that we track stock prices, economic indicators, and sports statistics.
Future Tense is a partnership of Slate, New America, and Arizona State University that examines emerging technologies, public policy, and society.
from Slate Magazine https://ift.tt/2xe566p
via IFTTT
沒有留言:
張貼留言