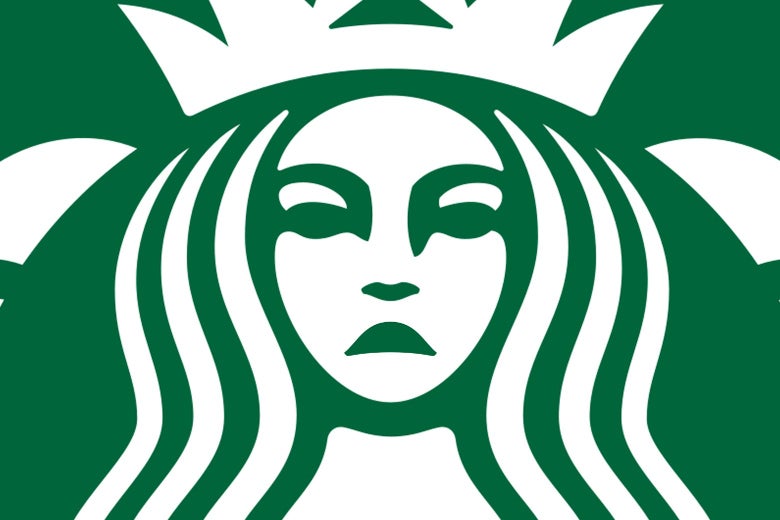
Photo illustration by Slate. Photo by Starbucks.
On Wednesday morning, Bloomberg reported some positive Starbucks news: The corporation had voluntarily disclosed a set of statistics on gender and racial pay equity. The numbers, I was pleasantly surprised to see, show no pay discrepancy between men and women, and also no differences along racial lines (no wonder they voluntarily disclosed them). That’s great, but there is some reason to be skeptical.
As a professional data scientist and an amateur pedant, I’m concerned that the metric Starbucks is using to measure that pay gap, the median, is imperfect for this type of analysis, to the point that it might smooth over real pay equity discrepancies because of the way it is calculated. When Starbucks disclosed its U.K. pay gap (as required by U.K. law), it had to report out both median and mean—those data showed a 5 percent gender discrepancy (favoring men). That’s still certainly better than the average company but is mathematically (I checked) a larger discrepancy than 0. Why does using the mean instead of the median show a greater discrepancy, and which one should you trust? Come along, dear reader, and we’ll learn all about measures of central tendency.
According to Laerd Statistics, “a measure of central tendency is a single value that attempts to describe a set of data by identifying the central position within that set of data.” Mean, median, and mode are measures of central tendency and are the three most used metrics in statistics (and are widely taught in U.S. schools—you surely remember this). They’re useful for quickly comparing two or more sets of information based on the “average” experience in that data set. Each of these three metrics can be a powerful decision-making tool, but they all have ideal use cases and can present significant drawbacks or obscure the truth when used improperly.
Assessing pay discrepancies requires looking at a big set of numbers to see if, on average, men are paid more than women, or white people are paid more than people of color, etc. To make this assessment, you’re not going to use the mode (the salary most people have); for Starbucks, the modal employee salary is certainly at or close to the entry-level wage. You’re going to want to use the median or the mean. The median is the “middle” value that separates a data set in half, with the higher values on one end and the lower values on the other end. So when Starbucks delivers its data based on the median and says there’s no discrepancy, what the company is really saying is the man who makes the middle amount of all men makes the same amount as the woman who makes the middle amount of all women. Is this proof of no pay discrepancies? Maybe. But it’s not that convincing. We really want to use mean, because that will allow us to unpack discrepancies for higher-wage workers or to look at discrepancies that might compound over time—say, for employees with shared titles where salaries might diverge over time even if the median salary is fixed at entry level.
Some analyses intentionally avoid the use of mean because outliers (very high values) can skew the data. For example, if you included the CEO’s pay alongside all the other workers, you could get an arbitrarily high value for average salary. But I’m curious to know what Starbucks’ mean numbers look like for the U.S. because while mean is subject to skew, it’s also the only measure that changes in value if any one data point increases or decreases. In fact, the data set Starbucks is analyzing necessitates the use of mean because of the many different factors that can make pay unequal.
The “modal” and “median” Starbucks employee is almost certainly an hourly worker who is hired at a set entry-level rate and who doesn’t negotiate compensation, raises, etc. This worker’s hiring managers are also unlikely to have real discretion over individual worker compensation, which means that there wouldn’t be meaningful pay equity problems for the vast majority of Starbucks employees. This would create median statistics that appear equal, even if a discrepancy exists higher up the chain. An even starting salary for managers, for example, could produce a seemingly equal median statistic if true gender gaps start to appear when individuals are promoted or given raises.
To see this, here’s an imaginary data set that visualizes hourly pay for 400 employees, broken out by gender, that could approximate workers at a coffee chain:
Mark Morgioni
In this data set, the median wage (the middle value that splits the data set in half) is $8 per hour for both men and women; if you were just looking at median, you would say that this imaginary company has indeed achieved pay equity. However, the mean hourly wage (the sum of all values divided by the total number of values) is $47.98 for men and $37.58 for women, which means pay for men is, on average, 27 percent higher than pay for women. That’s a big pay gap!
In order to truly decide that Starbucks has achieved a 0 percent gender and racial pay gap, we would need to see mean statistics. I reached out to Starbucks to ask for mean pay equity statistics, but the company hasn’t replied to my request (I’ll update if they do). Perhaps we should just remember to be skeptical of corporations that voluntarily disclose good pay equity numbers without providing a wider range of data. As a data scientist, I’m always wary of relying too heavily on a single statistic and am skeptical of those who present an unnuanced single source of truth.
from Slate Magazine https://ift.tt/2sHvX8e
via IFTTT
沒有留言:
張貼留言